Tidy Data with Pandas
Last updated on 2024-06-14 | Edit this page
Overview
Questions
- What are the benefits of transforming data into a tidy format for analysis?
- How does the melt() function in pandas facilitate data tidying?
- What are some practical challenges when working with real-world datasets in Python, and how can they be addressed?
Objectives
- Identify the characteristics of tidy data and explain its benefits, listing the three principles and discussing how it facilitates data analysis during a review session.
- Use pandas functions like concat(), melt(), and data filtering to manipulate and clean a complex dataset, successfully combining multiple files into a single DataFrame and reshaping it using melt()
Tidy Data in Pandas
Let’s import the pickle file that contains all of our Chicago public
library circulation data in a single DataFrame. We can use the Pandas
.read_pickle()
method to do so.
branch | address | city | zip code | january | february | march | april | may | june | july | august | september | october | november | december | ytd | year | |
---|---|---|---|---|---|---|---|---|---|---|---|---|---|---|---|---|---|---|
0 | Albany Park | 5150 N. Kimball Ave. | Chicago | 60625.0 | 8427 | 7023 | 9702 | 9344 | 8865 | 11650 | 11778 | 11306 | 10466 | 10997 | 10567 | 9934 | 120059 | 2011 |
1 | Altgeld | 13281 S. Corliss Ave. | Chicago | 60827.0 | 1258 | 708 | 854 | 804 | 816 | 870 | 713 | 480 | 702 | 927 | 787 | 692 | 9611 | 2011 |
2 | Archer Heights | 5055 S. Archer Ave. | Chicago | 60632.0 | 8104 | 6899 | 9329 | 9124 | 7472 | 8314 | 8116 | 9177 | 9033 | 9709 | 8809 | 7865 | 101951 | 2011 |
3 | Austin | 5615 W. Race Ave. | Chicago | 60644.0 | 1755 | 1316 | 1942 | 2200 | 2133 | 2359 | 2080 | 2405 | 2417 | 2571 | 2233 | 2116 | 25527 | 2011 |
4 | Austin-Irving | 6100 W. Irving Park Rd. | Chicago | 60634.0 | 12593 | 11791 | 14807 | 14382 | 11754 | 14402 | 14605 | 15164 | 14306 | 15357 | 14069 | 12404 | 165634 | 2011 |
branch | address | city | zip code | january | february | march | april | may | june | july | august | september | october | november | december | ytd | year | |
---|---|---|---|---|---|---|---|---|---|---|---|---|---|---|---|---|---|---|
958 | Chinatown | 2100 S. Wentworth Ave. | Chicago | 60616.0 | 4795 | 4258 | 5316 | 5343 | 4791 | 5367 | 5477 | 5362 | 4991 | 4847 | 4035 | 3957 | 58539 | 2022 |
959 | Brainerd | 1350 W. 89th St. | Chicago | 60620.0 | 255 | 264 | 370 | 386 | 399 | 421 | 337 | 373 | 361 | 276 | 256 | 201 | 3899 | 2022 |
960 | Brighton Park | 4314 S. Archer Ave. | Chicago | 60632.0 | 1394 | 1321 | 1327 | 1705 | 1609 | 1578 | 1609 | 1512 | 1425 | 1603 | 1579 | 1278 | 17940 | 2022 |
961 | South Chicago | 9055 S. Houston Ave. | Chicago | 60617.0 | 496 | 528 | 739 | 775 | 587 | 804 | 720 | 883 | 681 | 697 | 799 | 615 | 8324 | 2022 |
962 | Chicago Bee | 3647 S. State St. | Chicago | 60609.0 | 799 | 543 | 709 | 803 | 707 | 931 | 778 | 770 | 714 | 835 | 718 | 788 | 9095 | 2022 |
Let’s take a moment to discuss the setup of our DataFrame. It is structured in what is known as a wide format. This format displays an extensive amount of data directly on the screen, with each month’s circulation counts spread across the columns in a pivoted manner. This layout makes it easier to read and manually manipulate the data in a spreadsheet and because of this, is often the default output for periodic reporting systems like integrated library systems.
However, this wide format can pose challenges when working with data analysis tools like Pandas. For instance, if we need to identify all the library branches where circulation exceeded 10,000 in any given month, we would have to individually check each column dedicated to a month, which can be quite cumbersome.
To address this we can reshape our data in a long format. This is sometimes called un-pivoting the data, and in our case the month columns will become a single variable in the dataset.
Tidy Data
Tidy data is a standard way of organizing data values within a dataset, making it easier to work with. Here are the key principles of tidy data: 1. Every column holds a single variable, like “month” or “temperature.” 2. Every row represents a single observation, like circulation counts by branch and month. 3. Every cell contains a single value.
The image below might help orient us to the concept of tidy data.
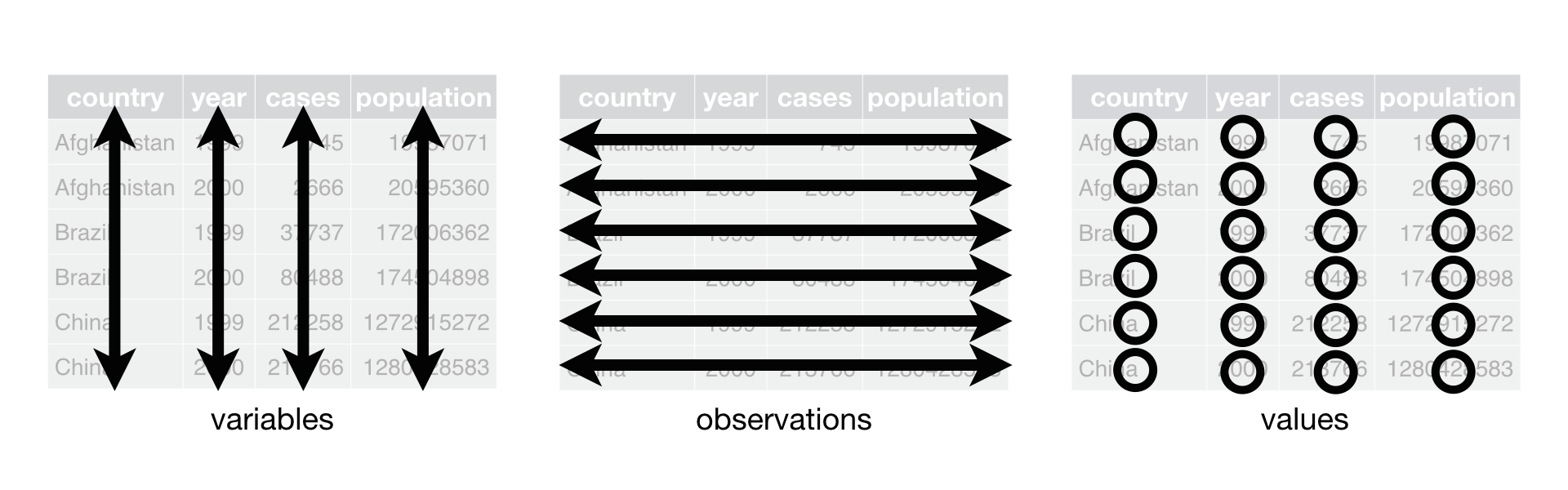
R for Data Science 12.1
Benefits of Tidy Data
Transforming our data into a tidy data format provides several advantages: - Python operations, such as visualization, filtering, and statistical analysis libraries, work better with data in a tidy format. - Tidy data makes transforming, summarizing, and visualizing information easier. For instance, comparing monthly trends or calculating annual averages becomes more straightforward. - As datasets grow, tidy data ensures that they remain manageable and analyses remain accurate.
Making Our Data Tidy
A good step towards tidying our data would be to consolidate the
separate month columns into a column called month,
and the
circulation counts into another column called
circulation_counts.
This simplifies our data and aligns
with the principles of tidy data.
To achieve this transformation, we can use a Pandas function called
melt()
. This function reshapes the data from wide to long
format, where each row will represent one month’s circulation data for a
branch. Let’s look at the help for melt
first.
Now, let’s tidy our data. We’ll create a new dataframe called
df_long
and use melt
to reshape.
melt
essentially melts down our columns into rows.
PYTHON
df_long = df.melt(id_vars=['branch', 'address', 'city', 'zip code', 'ytd', 'year'],
value_vars=['january', 'february', 'march', 'april', 'may', 'june',
'july', 'august', 'september', 'october', 'november', 'december'],
var_name='month', value_name='circulation')
In the above code we use id_vars
to list the columns we
do not want to melt. We then identify the columns we do want to melt
into rows in the value_vars
parameter.
var_name
is the variable name for the columns that will be
transformed into rows. value_names
is the measured
variable, circulation
in our case. Let’s now look at the
new structure of our data.
branch | address | city | zip code | ytd | year | month | circulation | |
---|---|---|---|---|---|---|---|---|
0 | Albany Park | 5150 N. Kimball Ave. | Chicago | 60625.0 | 120059 | 2011 | january | 8427 |
1 | Altgeld | 13281 S. Corliss Ave. | Chicago | 60827.0 | 9611 | 2011 | january | 1258 |
2 | Archer Heights | 5055 S. Archer Ave. | Chicago | 60632.0 | 101951 | 2011 | january | 8104 |
3 | Austin | 5615 W. Race Ave. | Chicago | 60644.0 | 25527 | 2011 | january | 1755 |
4 | Austin-Irving | 6100 W. Irving Park Rd. | Chicago | 60634.0 | 165634 | 2011 | january | 12593 |
… | … | … | … | … | … | … | … | … |
11551 | Chinatown | 2100 S. Wentworth Ave. | Chicago | 60616.0 | 58539 | 2022 | december | 3957 |
11552 | Brainerd | 1350 W. 89th St. | Chicago | 60620.0 | 3899 | 2022 | december | 201 |
11553 | Brighton Park | 4314 S. Archer Ave. | Chicago | 60632.0 | 17940 | 2022 | december | 1278 |
11554 | South Chicago | 9055 S. Houston Ave. | Chicago | 60617.0 | 8324 | 2022 | december | 615 |
11555 | Chicago Bee | 3647 S. State St. | Chicago | 60609.0 | 9095 | 2022 | december | 788 |
Ok, let’s look at the unique branches in our long DataFrame:
OUTPUT
array(['Albany Park', 'Altgeld', 'Archer Heights', 'Austin',
'Austin-Irving', 'Avalon', 'Back of the Yards', 'Beverly',
'Bezazian', 'Blackstone', 'Brainerd', 'Brighton Park',
'Bucktown-Wicker Park', 'Budlong Woods', 'Canaryville',
'Chicago Bee', 'Chicago Lawn', 'Chinatown', 'Clearing', 'Coleman',
'Daley, Richard J. - Bridgeport', 'Daley, Richard M. - W Humboldt',
'Douglass', 'Dunning', 'Edgebrook', 'Edgewater', 'Gage Park',
'Galewood-Mont Clare', 'Garfield Ridge', 'Greater Grand Crossing',
'Hall', 'Harold Washington Library Center', 'Hegewisch',
'Humboldt Park', 'Independence', 'Jefferson Park', 'Jeffery Manor',
'Kelly', 'King', 'Legler Regional', 'Lincoln Belmont',
'Lincoln Park', 'Little Village', 'Logan Square', 'Lozano',
'Manning', 'Mayfair', 'McKinley Park', 'Merlo', 'Mount Greenwood',
'Near North', 'North Austin', 'North Pulaski', 'Northtown',
'Oriole Park', 'Portage-Cragin', 'Pullman', 'Roden', 'Rogers Park',
'Roosevelt', 'Scottsdale', 'Sherman Park', 'South Chicago',
'South Shore', 'Sulzer Regional', 'Thurgood Marshall', 'Toman',
'Uptown', 'Vodak-East Side', 'Walker', 'Water Works',
'West Belmont', 'West Chicago Avenue', 'West Englewood',
'West Lawn', 'West Pullman', 'West Town', 'Whitney M. Young, Jr.',
'Woodson Regional', 'Wrightwood-Ashburn', 'Little Italy',
'West Loop'], dtype=object)
Alright! Now that we have the data tidied what can we do with it?
Let’s look at which branches circulated over 10,000 items in any given
month. We can filter the df_long
DataFrame to only show
rows that have a number greater than 10,000 in the
circulation
column.
branch | address | city | zip code | ytd | year | month | circulation | |
---|---|---|---|---|---|---|---|---|
4 | Austin-Irving | 6100 W. Irving Park Rd. | Chicago | 60634.0 | 165634 | 2011 | january | 12593 |
12 | Bucktown-Wicker Park | 1701 N. Milwaukee Ave. | Chicago | 60647.0 | 173396 | 2011 | january | 13113 |
13 | Budlong Woods | 5630 N. Lincoln Ave. | Chicago | 60659.0 | 160271 | 2011 | january | 12841 |
17 | Chinatown | 2353 S. Wentworth Ave. | Chicago | 60616.0 | 158449 | 2011 | january | 14027 |
24 | Edgebrook | 5331 W. Devon Ave. | Chicago | 60646.0 | 129288 | 2011 | january | 10231 |
… | … | … | … | … | … | … | … | … |
11373 | Harold Washington Library Center | 400 S. State St. | Chicago | 60605.0 | 276878 | 2020 | december | 20990 |
11420 | Sulzer Regional | 4455 N. Lincoln Ave. | Chicago | 60625.0 | 260163 | 2021 | december | 21671 |
11454 | Harold Washington Library Center | 400 S. State St. | Chicago | 60605.0 | 271811 | 2021 | december | 21046 |
11532 | Harold Washington Library Center | 400 S. State St. | Chicago | 60605.0 | 273406 | 2022 | december | 20480 |
11545 | Sulzer Regional | 4455 N. Lincoln Ave. | Chicago | 60625.0 | 301340 | 2022 | december | 21258 |
1434 rows × 8 columns
We can look at specific columns:
branch | circulation | |
---|---|---|
0 | Albany Park | 8427 |
1 | Altgeld | 1258 |
2 | Archer Heights | 8104 |
3 | Austin | 1755 |
4 | Austin-Irving | 12593 |
… | … | … |
11551 | Chinatown | 3957 |
11552 | Brainerd | 201 |
11553 | Brighton Park | 1278 |
11554 | South Chicago | 615 |
11555 | Chicago Bee | 788 |
11556 rows × 2 columns
We can sort our table using .sort_values()
to see the
branches with the highest circulation per month:
branch | address | city | zip code | ytd | year | month | circulation | |
---|---|---|---|---|---|---|---|---|
1957 | Harold Washington Library Center | 400 S. State St. | Chicago | 60605.0 | 966720 | 2011 | march | 89122 |
2920 | Harold Washington Library Center | 400 S. State St. | Chicago | 60605.0 | 966720 | 2011 | april | 88527 |
2999 | Harold Washington Library Center | 400 S. State St. | Chicago | 60605.0 | 937649 | 2012 | april | 87689 |
6772 | Harold Washington Library Center | 400 S. State St. | Chicago | 60605.0 | 966720 | 2011 | august | 85193 |
2036 | Harold Washington Library Center | 400 S. State St. | Chicago | 60605.0 | 937649 | 2012 | march | 84255 |
… | … | … | … | … | … | … | … | … |
3623 | Portage-Cragin | 5108 W. Belmont Ave. | Chicago | 60641.0 | 36262 | 2020 | april | 0 |
3622 | Manning | 6 S. Hoyne Ave. | Chicago | 60612.0 | 3325 | 2020 | april | 0 |
3621 | Daley, Richard J. - Bridgeport | 3400 S. Halsted St. | Chicago | 60608.0 | 37045 | 2020 | april | 0 |
3620 | Canaryville | 642 W. 43rd St. | Chicago | 60609.0 | 4120 | 2020 | april | 0 |
3577 | Merlo | 644 W. Belmont Ave. | Chicago | 60657.0 | 14637 | 2019 | april | 0 |
11556 rows × 8 columns
What if we want to tally up the total circulation for each branch over all years and also see the mean circulation?
PYTHON
df_long.groupby('branch')['circulation'].agg(total_circulation='sum', mean_circulation='mean')
total_circulation | mean_circulation | |
---|---|---|
branch | ||
Albany Park | 1024714 | 7116.069444 |
Altgeld | 68358 | 474.708333 |
Archer Heights | 803014 | 5576.486111 |
Austin | 200107 | 1389.631944 |
Austin-Irving | 1359700 | 9442.361111 |
… | … | … |
West Pullman | 295327 | 2050.881944 |
West Town | 922876 | 6408.861111 |
Whitney M. Young, Jr. | 259680 | 1803.333333 |
Woodson Regional | 823793 | 5720.784722 |
Wrightwood-Ashburn | 302285 | 2099.201389 |
82 rows × 2 columns
-
df.groupby('branch')
: This groups the data by the ‘branch’ column so that all entries in the DataFrame with the same library branch are grouped together. (This is similar to the SQLGROUP BY
statement or thegroup_by
function indplyr
in R.) -
['circulation']
: After grouping the data by branch, this specifies that subsequent operations should be performed on the ‘circulation’ column. -
.agg(...)
: Theagg
function is used to apply one or more aggregation operations to the grouped data. Inside theagg
function:-
total_circulation='sum'
: This creates a new column named ‘total_circulation’ where each entry is the sum of ‘circulation’ for that branch. It totals up all circulation figures within each branch. -
mean_circulation='mean'
: This creates a new column named ‘mean_circulation’ where each entry is the average ‘circulation’ for that branch. It calculates the mean circulation figures for each branch.
-
If we want to group by more than one variable, we can list those
column names in the .groupby()
function.
sum | mean | ||
---|---|---|---|
branch | month | ||
Albany Park | april | 79599 | 6633.250000 |
august | 91416 | 7618.000000 | |
december | 77849 | 6487.416667 | |
february | 76747 | 6395.583333 | |
january | 85952 | 7162.666667 | |
… | … | … | … |
Wrightwood-Ashburn | march | 25817 | 2151.416667 |
may | 22049 | 1837.416667 | |
november | 24124 | 2010.333333 | |
october | 27345 | 2278.750000 | |
september | 25692 | 2141.000000 |
984 rows × 2 columns
Adding a Date Column
In order to plot this data over time in the data visualization we
need to do three things to prepare it. First, we need to combine the
year and month columns into its own column. Second, convert the new date
column to a datetime
objec using the Pandas to_datetime
function. Third, we
assign the date column as our index for the data. These steps will set
up our data for plotting.
This will create a new column in our data frame by adding our year
and month together separated by a -
. This setup is not
sufficient for us to use .to_datetime()
to convert the
column to something Python and Pandas knows is a date.
pd.to_datetime()
will do the conversion, but we need to
tell it how we have our date formatted. In this case we have year and
month name spelled out. To find more format codes, see https://docs.python.org/3/library/datetime.html#strftime-and-strptime-behavior.
If we take a look at the date column, we’ll see that datetime
automatically adds a day (always 01
) in the absence of any
specific day input.
OUTPUT
0 2011-01-01
1 2011-01-01
2 2011-01-01
3 2011-01-01
4 2011-01-01
...
11551 2022-12-01
11552 2022-12-01
11553 2022-12-01
11554 2022-12-01
11555 2022-12-01
Name: date, Length: 11556, dtype: datetime64[ns]
OUTPUT
<class 'pandas.core.frame.DataFrame'>
RangeIndex: 11556 entries, 0 to 11555
Data columns (total 9 columns):
# Column Non-Null Count Dtype
--- ------ -------------- -----
0 branch 11556 non-null object
1 address 7716 non-null object
2 city 7716 non-null object
3 zip code 7716 non-null float64
4 ytd 11556 non-null int64
5 year 11556 non-null object
6 month 11556 non-null object
7 circulation 11556 non-null int64
8 date 11556 non-null datetime64[ns]
dtypes: datetime64[ns](1), float64(1), int64(2), object(5)
memory usage: 812.7+ KB
That worked! Now, we can make the datetime column the index of our
DataFrame. In the Pandas episode we looked at Pandas default numerical
index, but we can also use .set_index()
to declare a
specific column as the index of our DataFrame. Using a datetime index
will make it easier for us to plot the DataFrame over time. The first
parameter of .set_index()
is the column name and the
inplace=True
parameter allows us to modify the DataFrame
without assigning it to a new variable.
If we look at the data again, we will see our index will be set to date.
Let’s save df_long
to use in the next episode.
Tidy Data Principles
How would you reorganize the following table about research data workshops to follow the three tidy data principles?
- Every column holds a single variable.
- Every row represents a single observation.
- Every cell contains a single value.
Date | Length | Content | Instructor |
---|---|---|---|
2023-01-15 | 30 min | RDM, DMP | CH |
2023-02-02 | 2 hours | Python, RDM | CH, TD |
2023-02-03 | 90 min | Python | SP |
You can use each content unit (e.g., RDM, DMP, Python) as an observation, and breakdown the length of time or instructor initials to match the content unit however you like.
Year | Month | Day | Length (min) | Content | Instructor |
---|---|---|---|---|---|
2023 | 01 | 15 | 20 | RDM | CH |
2023 | 01 | 15 | 10 | DMP | CH |
2023 | 02 | 02 | 100 | Python | TD |
2023 | 02 | 02 | 20 | RDM | CH |
2023 | 02 | 03 | 100 | Python | SP |
Subsetting df_long
Using df_long, create a new DataFrame, `low_circ’, that only includes branches with circulation numbers lower than 500 per month. When you create a subset DataFrame, show the following columns: branch, circulation, month, and year. Next, eliminate the rows when the circulation is equal to 0.
Group and aggregate for circulation by year
How would you create a subset of df_long
that sums up
the circulation by year across all branches? In other words you want a
view of the DataFrame that includes one row for each year, and columns
for ‘year’ and ‘sum’, the latter of which shows the sum of circulation
for all branches in each year.